Detect Your Fingerprint in Your Photographs: Photography-based Multi-Feature Sybil Detection
Authors: Yerim Kim (Korea University), Minjae Kim (Korea University), Myungjae Chung (Korea University), Junbeom Hur (Korea University)
Volume: 2023
Issue: 1
Pages: 244–263
DOI: https://doi.org/10.56553/popets-2023-0015
Abstract: A darknet market is an online marketplace typically implemented over Tor, where vendors sell illegal products or criminal services. Due to dramatic growth in the popularity of such markets, there is a recognized need for automatic investigation of the market’s ecosystem and identification of anonymous vendors. However, as they often create multiple accounts (or Sybil accounts) within or across different marketplaces, detecting Sybil accounts becomes the key to understanding the ecosystem of darknet markets and identifying the actual relationship between the vendors. This study presents a novel Sybil detection method that extracts multiple features of vendors from photographs in a fine-grained level (e.g., image similarity, main category, subcategory, and text data), and reveals the multiple Sybil accounts of them simultaneously. Each feature is extracted from multiple rich sources using an image hash algorithm, Deep Neural Network (DNN) classifier, image restoration, and text recognition tool; and merged using a weighted feature embedding model. The matching score of each vendor is then calculated to identify not only the exact Sybil accounts, but multiple potential accounts suspected of being associated to a single operator. We evaluate the efficacy of our method using real-world datasets from four large darknet markets (i.e., SilkRoad2, Agora, Evolution, Alphabay) from 2014 to 2015. Because of the anonymity of darknet market, we construct the ground-truth of Sybil accounts by randomly splitting the dataset of vendors into two even parts. We used the first set to train the model, and linked the second set to the original vendor in the first set to evaluate performance. Our experimental results demonstrated that the proposed method outperforms the existing photography-based system with an accuracy of 98%, identifying up to 700% more candidate Sybil accounts than prior work. Additionally, our method detects multiple Sybil accounts for 90% of evaluated test cases, presenting a very different picture of darknet marketplace dynamics than methods that can only detect a single Sybil account for each target vendor. Due to its fine-grained multiple feature extraction from photographs, our method can be more generically applied to various darknet markets for Sybil detection regardless of their languages or categories of items.
Keywords: Darkweb, Sybil detection, De-anonymization
Copyright in PoPETs articles are held by their authors. This article is published under a Creative Commons Attribution 4.0 license.
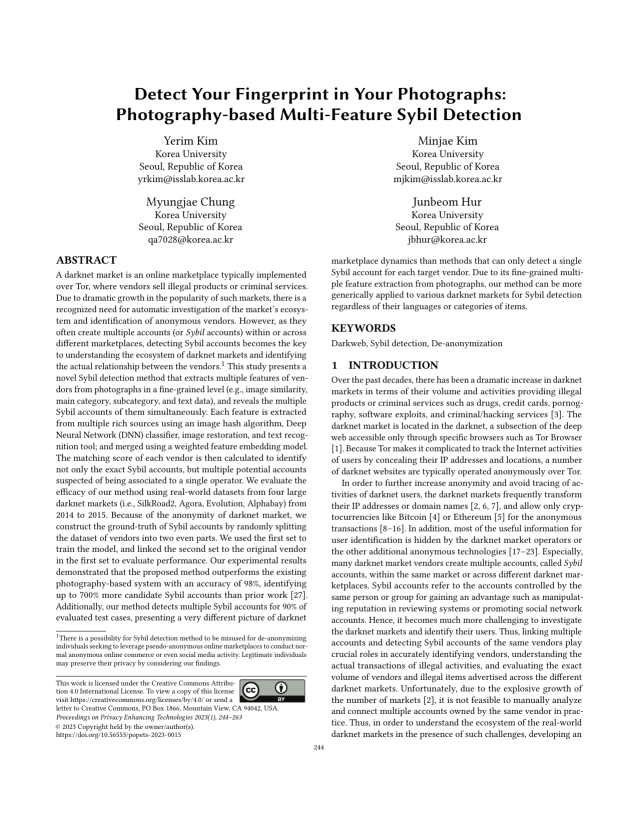