StyleID: Identity Disentanglement for Anonymizing Faces
Authors: Minh-Ha Le (Linköping University), Niklas Carlsson (Linköping University)
Volume: 2023
Issue: 1
Pages: 264–278
DOI: https://doi.org/10.56553/popets-2023-0016
Abstract: Privacy of machine learning models is one of the remaining challenges that hinder the broad adoption of Artificial Intelligent (AI). This paper considers this problem in the context of image datasets containing faces. Anonymization of such datasets is becoming increasingly important due to their central role in the training of autonomous cars, for example, and the vast amount of data generated by surveillance systems. While most prior work de-identifies facial images by modifying identity features in pixel space, we instead project the image onto the latent space of a Generative Adversarial Network (GAN) model, find the features that provide the biggest identity disentanglement, and then manipulate these features in latent space, pixel space, or both. The main contribution of the paper is the design of a feature-preserving anonymization framework, StyleID, which protects the individuals’ identity, while preserving as many characteristics of the original faces in the image dataset as possible. As part of the contribution, we present a novel disentanglement metric, three complementing disentanglement methods, and new insights into identity disentanglement. StyleID provides tunable privacy, has low computational complexity, and is shown to outperform current state-of-the-art solutions.
Keywords: identity disentanglement, anonymization, feature-preserving, privacy, StyleGAN, face editing
Copyright in PoPETs articles are held by their authors. This article is published under a Creative Commons Attribution 4.0 license.
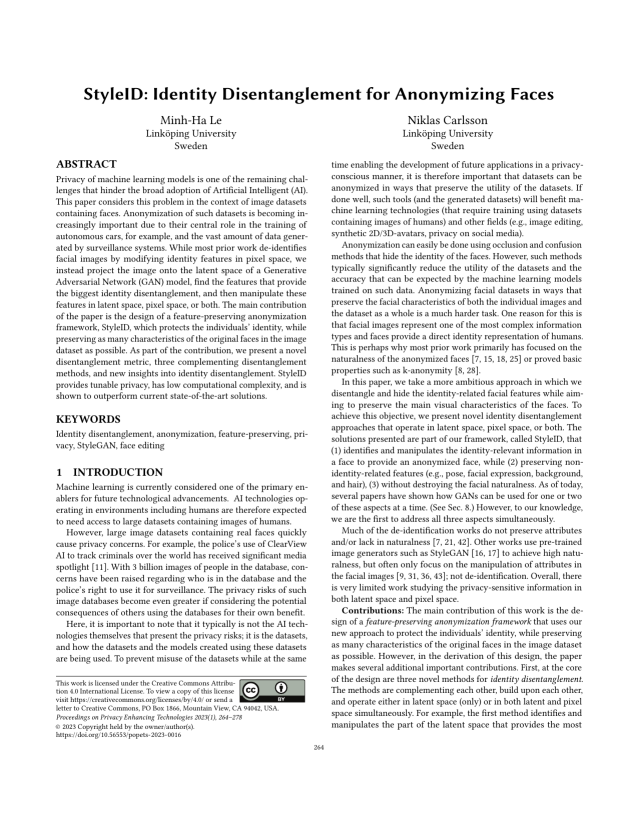