Privacy-Aware Adversarial Network in Human Mobility Prediction
Authors: Yuting Zhan (Imperial College London), Hamed Haddadi (Imperial College London), Afra Mashhadi (University of Washington)
Volume: 2023
Issue: 1
Pages: 556–570
DOI: https://doi.org/10.56553/popets-2023-0032
Abstract: As mobile devices and location-based services are increasingly developed in different smart city scenarios and applications, many unexpected privacy leakages have arisen due to geolocated data collection and sharing. User re-identification and other sensitive inferences are major privacy threats when geolocated data are shared with cloud-assisted applications. Significantly, four spatio-temporal points are enough to uniquely identify 95% of the individuals, which exacerbates personal information leakages. To tackle malicious purposes such as user re-identification, we propose an LSTM-based adversarial mechanism with representation learning to attain a privacy-preserving feature representation of the original geolocated data (i.e., mobility data) for a sharing purpose. These representations aim to maximally reduce the chance of user re-identification and full data reconstruction with a minimal utility budget (i.e., loss). We train the mechanism by quantifying privacy-utility trade-off of mobility datasets in terms of trajectory reconstruction risk, user re-identification risk, and mobility predictability. We report an exploratory analysis that enables the user to assess this trade-off with a specific loss function and its weight parameters. The extensive comparison results on four representative mobility datasets demonstrate the superiority of our proposed architecture in mobility privacy protection and the efficiency of the proposed privacy-preserving features extractor. We show that the privacy of mobility traces attains decent protection at the cost of marginal mobility utility. Our results also show that by exploring the Pareto optimal setting, we can simultaneously increase both privacy (45%) and utility (32%).
Keywords: LSTM neural networks, mobility prediction, data privacy, adversarial learning
Copyright in PoPETs articles are held by their authors. This article is published under a Creative Commons Attribution 4.0 license.
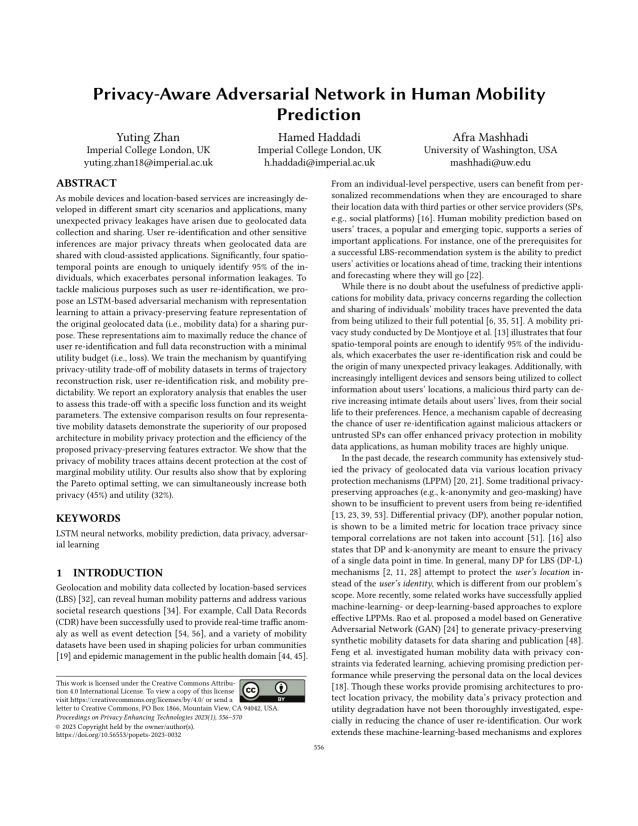