SoK: Managing risks of linkage attacks on data privacy
Authors: Jovan Powar (University of Cambridge), Alastair R. Beresford (University of Cambridge)
Volume: 2023
Issue: 2
Pages: 97–116
DOI: https://doi.org/10.56553/popets-2023-0043
Abstract: Novel attacks on dataset privacy are usually met with the same range of responses: surprise that a route to information gain exists from information previously thought to be safe; disputes around the viability or validity of the attack in real-world contexts; and, in the case of the computer science community, a drive to produce techniques that provably protect against the new class of attack. The result is a disjointed landscape with no shared approach to modelling threats to dataset privacy, and a toolbox of technically complex systems whose guarantees come with narrow assumptions and whose application in real-world contexts is hard to achieve. In this paper we aim to understand these issues by charting the history of dataset privacy attacks and systematising breaches through the lens of data linkage. We show how identification or information gain on a dataset's subjects can be expressed as data linkage, and use this to present a taxonomy of threat models which we apply to ninety-four attacks from across the literature. Our work demonstrates that dataset privacy must be approached first as a risk management problem, rather than one of strict guarantees, an approach which aligns well with law and practice. Our taxonomy of attacker intents provides a coherent language for expressing the wide variety of threat models in dataset privacy, and a framework for understanding how risks identified under one model can be understood within another. We also present insights around the factors that affect the feasibility and severity of attacks, and proposals for practical techniques that can be used for risk appraisal and management by practitioners, researchers, and regulators alike.
Keywords: data sharing, identification, reidentification, anonymity, risk appraisal, data protection, record linkage, membership inference, attribute inference, threat modelling
Copyright in PoPETs articles are held by their authors. This article is published under a Creative Commons Attribution 4.0 license.
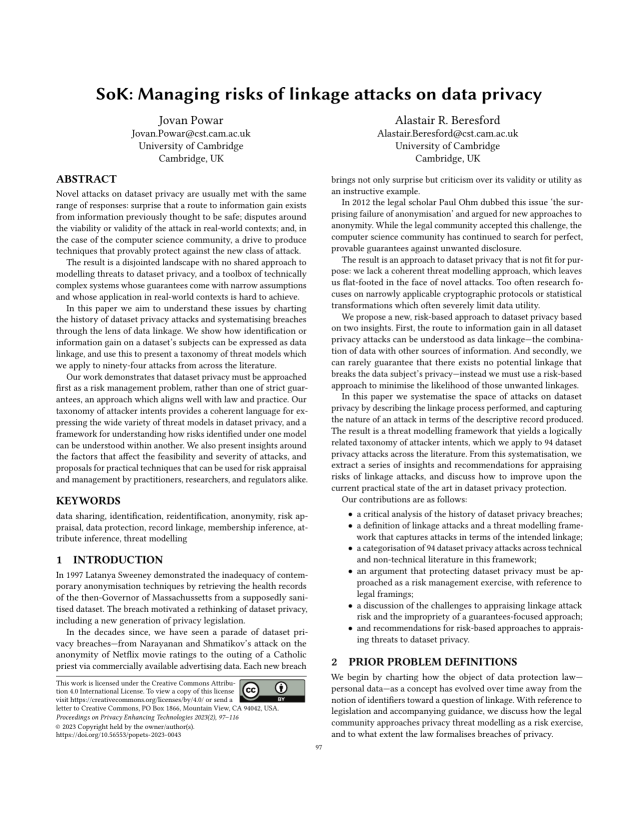