Distributed GAN-Based Privacy-Preserving Publication of Vertically-Partitioned Data
Authors: Xue Jiang (Technical University of Munich), Yufei Zhang (Technical University of Munich), Xuebing Zhou (Huawei Munich Research Center), Jens Grossklags (Technical University of Munich)
Volume: 2023
Issue: 2
Pages: 236–250
DOI: https://doi.org/10.56553/popets-2023-0050
Abstract: In the era of big data, user data are often vertically partitioned and stored at different local parties. Exploring the data from all the local parties would enable data analysts to gain a better understanding of the user population from different perspectives. However, the publication of vertically-partitioned data faces a dilemma: on the one hand, the original data cannot be directly shared by local parties due to privacy concerns; on the other hand, independently privatizing the local datasets before publishing may break the potential correlation between the cross-party attributes and lead to a significant utility loss. Prior solutions compute the privatized multivariate distributions of different attribute sets for constructing a synthetic integrated dataset. However, these algorithms are only applicable for low-dimensional structured data and may suffer from large utility loss with the increase in data dimensionality. Following the idea of synthetic data generation, we propose VertiGAN, the first framework based on a generative adversarial network (GAN) for publishing vertically-partitioned data with privacy protection. The framework adopts a GAN model comprised of one multi-output global generator and multiple local discriminators. The generator is collaboratively trained by the server and local parties to learn the distribution of all parties' local data and is used to generate a high-utility synthetic integrated dataset on the server side. Additionally, we apply differential privacy (DP) during the training process to ensure strict privacy guarantees for the local data. We evaluate the framework's performance on a number of real-world datasets containing 68--1501 classification attributes and show that our framework is more capable of capturing joint distributions and cross-attribute correlations compared to statistics-based baseline algorithms. Moreover, with a privacy guarantee of epsilon=8, our framework achieves around a 2%~15% improvement in classification accuracy compared to the baseline algorithms. Extensive experimental results demonstrate the capability and efficiency of our framework in synthesizing vertically-partitioned data while striking a satisfactory utility-privacy balance.
Keywords: Differential privacy, vertically-partitioned data, synthetic data
Copyright in PoPETs articles are held by their authors. This article is published under a Creative Commons Attribution 4.0 license.
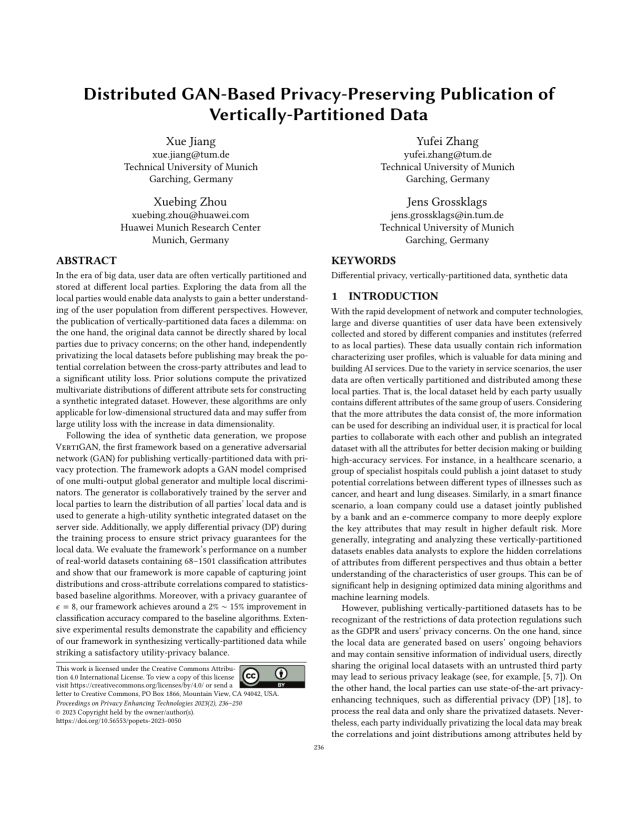