SoK: Differentially Private Publication of Trajectory Data
Authors: Àlex Miranda-Pascual (Universitat Politècnica de Catalunya and Karlsruhe Institute of Technology), Patricia Guerra-Balboa (Karlsruhe Institute of Technology), Javier Parra-Arnau (Universitat Politècnica de Catalunya and Karlsruhe Institute of Technology), Jordi Forné (Universitat Politècnica de Catalunya), Thorsten Strufe (Karlsruhe Institute of Technology)
Volume: 2023
Issue: 2
Pages: 496–516
DOI: https://doi.org/10.56553/popets-2023-0065
Abstract: Trajectory analysis holds many promises, from improvements in traffic management to routing advice or infrastructure development. However, learning users' paths is extremely privacy-invasive. Therefore, there is a necessity to protect trajectories such that we preserve the global properties, useful for analysis, while specific and private information of individuals remains inaccessible. Trajectories, however, are difficult to protect, since they are sequential, highly dimensional, correlated, bound to geophysical restrictions, and easily mapped to semantic points of interest. This paper aims to establish a systematic framework on protective masking and synthetic-generation measures for trajectory databases with syntactic and differentially private (DP) guarantees, including also utility properties, derived from ideas and limitations of existing proposals. To reach this goal, we systematize the utility metrics used throughout the literature, deeply analyze the DP granularity notions, explore and elaborate on the state of the art on privacy-enhancing mechanisms and their problems, and expose the main limitations of DP notions in the context of trajectories.
Keywords: systematization of knowledge, privacy-preserving data publishing, trajectory privacy, differential privacy, utility metrics
Copyright in PoPETs articles are held by their authors. This article is published under a Creative Commons Attribution 4.0 license.
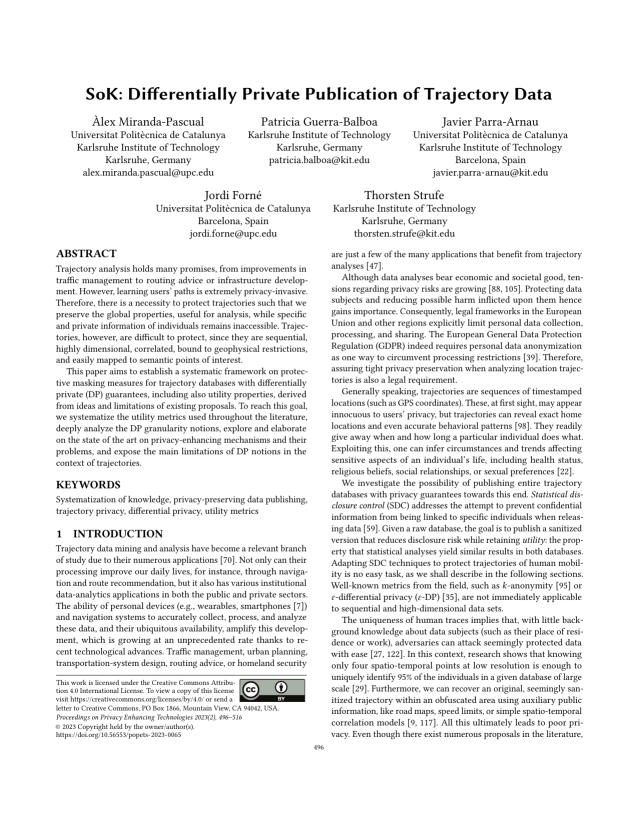