Towards Sentence Level Inference Attack Against Pre-trained Language Models
Authors: Kang Gu (Dartmouth College), Ehsanul Kabir (Penn State University), Neha Ramsurrun (Dartmouth College), Soroush Vosoughi (Dartmouth College), Shagufta Mehnaz (Penn State University)
Volume: 2023
Issue: 3
Pages: 62–78
DOI: https://doi.org/10.56553/popets-2023-0070
Abstract: In recent years, pre-trained language models (e.g., BERT and GPT) have shown the superior capability of textual representation learning, benefiting from their large architectures and massive training corpora. The industry has also quickly embraced language models to develop various downstream NLP applications. For example, Google has already used BERT to improve its search system. The utility of the language embeddings also brings about potential privacy risks. Prior works have revealed that an adversary can either identify whether a keyword exists or gather a set of possible candidates for each word in a sentence embedding. However, these attacks cannot recover coherent sentences which leak high-level semantic information from the original text. To demonstrate that the adversary can go beyond the word-level attack, we present a novel decoder-based attack, which can reconstruct meaningful text from private embeddings after being pre-trained on a public dataset of the same domain. This attack is more challenging than a word-level attack due to the complexity of sentence structures. We comprehensively evaluate our attack in two domains and with different settings to show its superiority over the baseline attacks. Quantitative experimental results show that our attack can identify up to 3.5X of the number of keywords identified by the baseline attacks. Although our method reconstructs high-quality sentences in many cases, it often produces lower-quality sentences as well. We discuss these cases and the limitations of our method in detail
Keywords: Pre-trained Language Models, Inference Attack, Text Reconstruction
Copyright in PoPETs articles are held by their authors. This article is published under a Creative Commons Attribution 4.0 license.
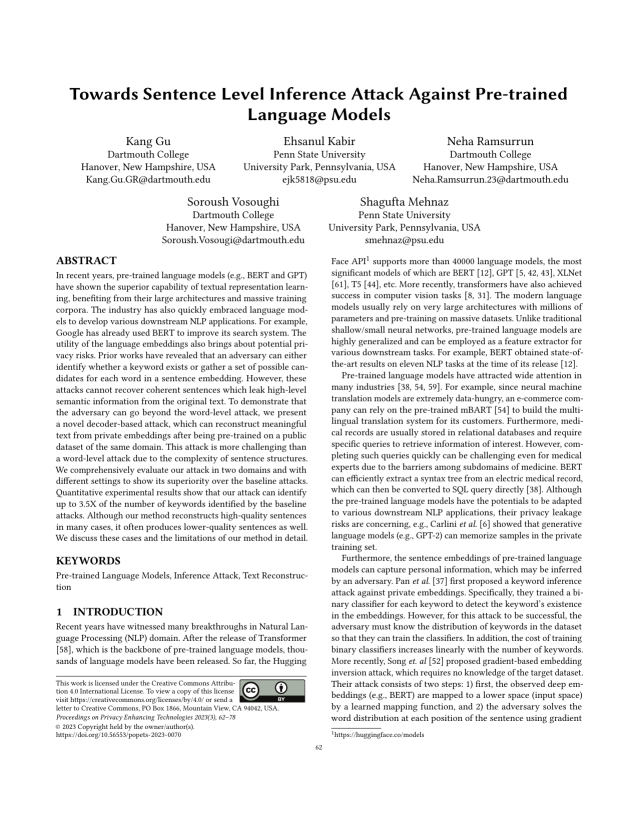