SenRev: Measurement of Personal Information Disclosure in Online Health Communities
Authors: Faysal Hossain Shezan (University of Virginia), Minjun Long (University of Virginia), David Hasani (University of Virginia), Gang Wang (University of Illinois at Urbana-Champaign), Yuan Tian (University of California Los Angeles)
Volume: 2023
Issue: 3
Pages: 233–251
DOI: https://doi.org/10.56553/popets-2023-0079
Abstract: With life style shifting during the pandemic, online health communities start to attract more users (including healthcare workers and patients) to discuss health-related questions. While such online platforms provide convenience to users, with health-related information shared broadly over text and images (e.g., X-Ray scans, photocopies of documents), they also raise questions regarding privacy. In this paper, we propose SenRev to systematically measure the leakages of sensitive information in those publicly available discussions. We use SenRev to analyze 1,894,900 multi-modal and multi-lingual data elements from four different online health communities. We find that sensitive data leakages are common; overall 1,324,064 (69.88%) pieces of evidence of data leakages are detected, with 23,587 (1.78%) of them involving identifiers and 1,300,477 (98.22%) involving quasi-identifiers. Surprisingly, leakages through medical images occur more frequently in the community of healthcare professionals compared with the other communities. Finally, based on our results, we discuss the potential directions for countermeasures.
Keywords: Privacy, Personal Information Leakages, Online Health Communities
Copyright in PoPETs articles are held by their authors. This article is published under a Creative Commons Attribution 4.0 license.
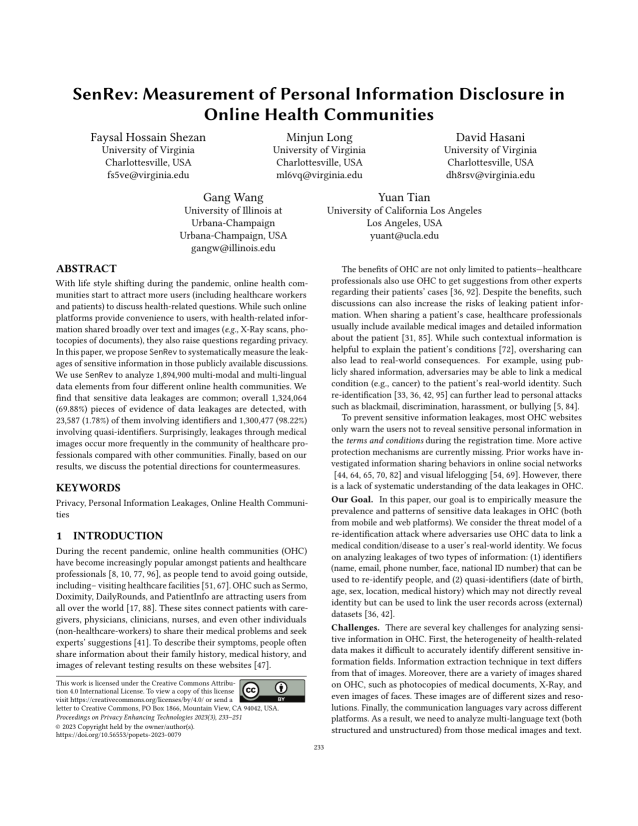