"My face, my rules": Enabling Personalized Protection Against Unacceptable Face Editing
Authors: Zhujun Xiao (University of Chicago), Jenna Cryan (University of Chicago), Yuanshun Yao (University of Chicago), Yi Hong Gordon Cheo (University of Chicago), Yuanchao Shu (Zhejiang University), Stefan Saroiu (Microsoft Research), Ben Y. Zhao (University of Chicago), Haitao Zheng (University of Chicago)
Volume: 2023
Issue: 3
Pages: 252–267
DOI: https://doi.org/10.56553/popets-2023-0080
Abstract: Today, face editing is widely used to refine/alter photos in both professional and recreational settings. Yet it is also used to modify (and repost) existing online photos for cyberbullying. Our work considers an important open question: 'How can we support the collaborative use of face editing on social platforms while protecting against unacceptable edits and reposts by others?' This is challenging because, as our user study shows, users vary widely in their definition of what edits are (un)acceptable. Any global filter policy deployed by social platforms is unlikely to address the needs of all users, but hinders social interactions enabled by photo editing. Instead, we argue that face edit protection policies should be implemented by social platforms based on individual user preferences. When posting an original photo online, a user can choose to specify the types of face edits (dis)allowed on the photo. Social platforms use these per-photo edit policies to moderate future photo uploads, i.e., edited photos containing modifications that violate the original photo's policy are either blocked or shelved for user approval. Realizing this personalized protection, however, faces two immediate challenges: (1) how to accurately recognize specific modifications, if any, contained in a photo; and (2) how to associate an edited photo with its original photo (and thus the edit policy). We show that these challenges can be addressed by combining highly efficient hashing based image search and scalable semantic image comparison, and build a prototype protector (Alethia) covering nine edit types. Evaluations using IRB-approved user studies and data-driven experiments (on 839K face photos) show that Alethia accurately recognizes edited photos that violate user policies and induces a feeling of protection to study participants. This demonstrates the initial feasibility of personalized face edit protection. We also discuss current limitations and future directions to push the concept forward.
Keywords: face edit, personalized protection, image moderation
Copyright in PoPETs articles are held by their authors. This article is published under a Creative Commons Attribution 4.0 license.
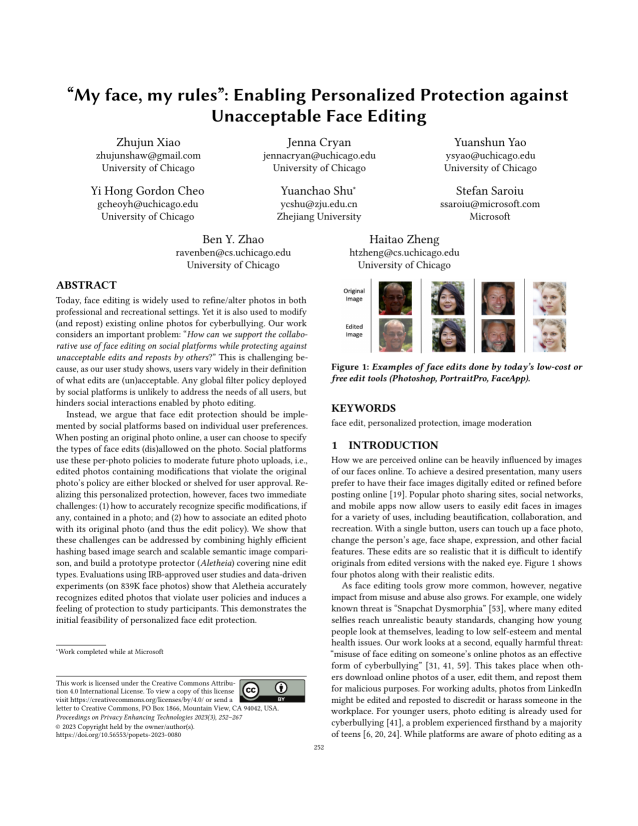